For many organizations, data migration is the first major challenge of data modernization, with the process often taking six to twelve months for larger companies as they make the leap from legacy technologies to a centralized data cloud platform.
A faster, more efficient migration process means organizations can begin to see returns on their data tech investments sooner while expediting their modernization journey and developing toward more data-mature practices like data monetization at a faster rate.
It’s no wonder that the “lift and shift” model of data migration has persisted so long, with organizations eager to migrate their data to their new cloud platform as quickly as possible and a host of SIs on the market offering to get the work done. But this traditional migration approach misses the mark, both in terms of immediate expediency and in laying the foundation for future data maturity and innovation.
Let’s take a look at how organizations can break free of the traditional approach to data migration in order to accelerate the migration process, push forward their modernization timelines, tap into the benefits of the modern data stack faster, and expedite ROI on their data tech investments.
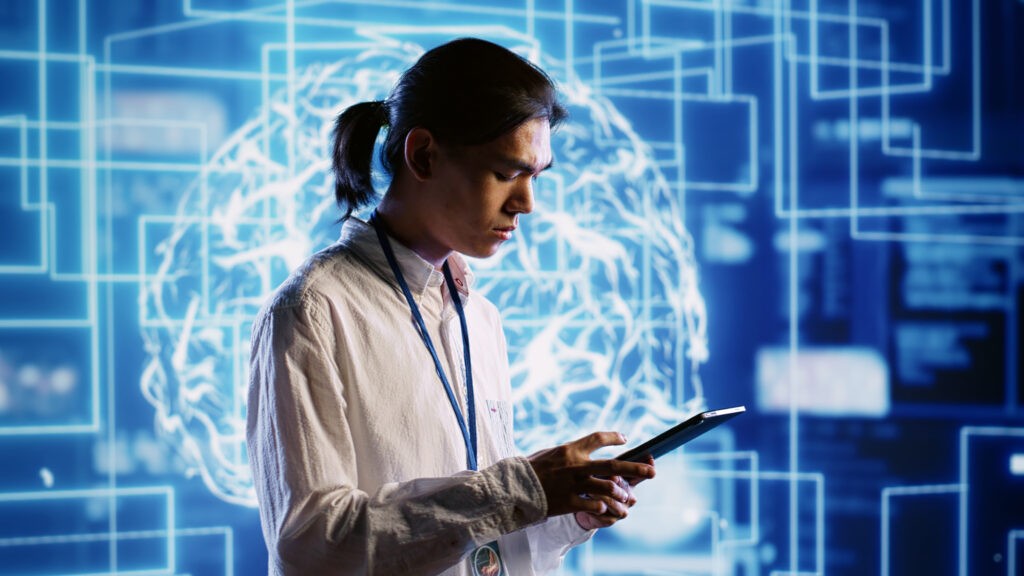
Improving on the Traditional Migration Approach
Traditional data migration processes rely heavily on human expertise and manual processing, which means these processes are necessarily limited by human capabilities. For every step in the migration process, a team of experts must have a deep understanding of how data is being transferred from one repository to another, with only minimal help from machine learning models to facilitate things.
But AI can now be deployed to do much of the heavy lifting throughout these processes, with AI copilots rationalizing ETL pipelines and AI generation being leveraged to build optimized cloud models. The stages, loads, and pipelines that make up the bulk of the migration process can now be expedited by automated DAGs, and the validation of pipelines and results can be similarly automated.
Traditionally, the conversion stage that follows the initial migration stage involves heavy use of SnowConvert or other similar tools specific to their respective vendors. The success of this stage depends on rationalizing an optimal data model before converting code and entities into this new model.
This process can be enhanced by external support from consultants like Hakkoda, whose deep industry insight and expertise in the Snowflake AI Data Cloud can ensure processes are carried out with scalability and optimization in mind. Here, experience with industry standards and conversion best practices are the keys to success, whereas a more traditional approach would rely almost exclusively on tools like SnowConvert, without giving appropriate attention to future data maturity potential.
In the final stage, when the new data model is in place and ready to be reconnected to organizational operations, the traditional model is once again limited by human ingenuity and manpower. Data engineers have to contend with complex development questions like “How do existing points of consumption get fed?” and “How will thousands of reports and applications be digested in order to determine what stays?” and so on.
A streamlined approach again takes the development burden off of human expertise by enlisting Gen AI and AI copilots to oversee everything from rationalizing reports and dashboards, to generating optimized analytical models. Human engineering is thus limited to optimizing these AI tools and driving L&D.
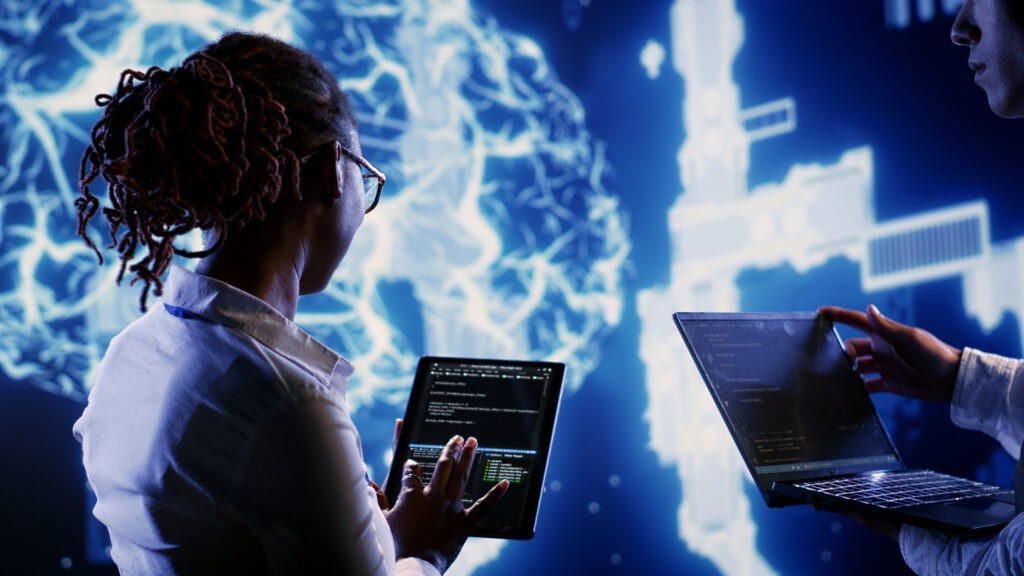
Data Migration is About So Much More than “Lifting and Shifting”
Improving on the traditional approach to data migration by leveraging sophisticated AI tools and thus cutting down on human labor is one of Hakkoda’s specialties. But it’s not just our expertise with powerful AI tools that distinguishes us as migration experts.
Say goodbye to the old “lift and shift” approach to migration, as our data migration service is much more than a one-time transfer to the cloud. Our approach is modeled after our Data Innovation Journey framework and is designed to see your organization grow from data chaos to data innovation.
That means that once the migration fundamentals are in place and validated, our experts continue to work with client teams to optimize their Snowflake environment and extract actionable insight from their data, building out reporting and analytics functionality along the way.
To help customers actualize the full potential of their modernized data stack after a successful migration, Hakkoda works closely with internal stakeholders to chart a course for innovation. This final stage in the data maturity journey includes game-changing, outcome-oriented use cases like AI integrations and direct data monetization on the Snowflake Marketplace.
But our long-term approach to setting up your data stack for success doesn’t mean slowing down your migration timeline—just the opposite, in fact. With our data migration service, organizations have 16 times the migration speed, thanks to Snowflake accelerators and Hakkoda IP. That means you’ll tap into the 60% cost reduction that comes with transitioning to Snowflake faster.
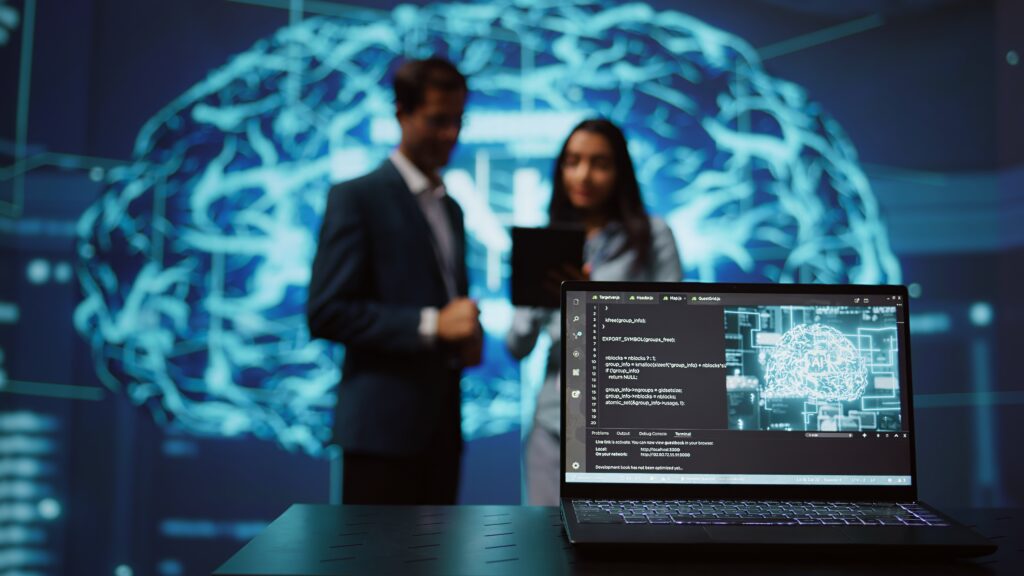
Data Migration that Sets Up Your Organization for Innovation
It’s no secret that data migration is a delicate and complex step in any organization’s data modernization journey. A flawed approach can mean months of wasted time and unrealized ROI, but even the “traditional” approach, followed to the letter, can leave your organization with a data stack that isn’t built to scale and that lacks the infrastructure to pave the way to greater data maturity.
If you’re ready to ditch the traditional “lift and shift” approach and optimize your company’s migration journey, tapping into the power of transformative AI tools to expedite processes while leveraging deep expertise to develop a forward-thinking data stack, let’s talk today about how Hakkoda can help your organization realize its data modernization goals.