The ability to run performant, cost-effective analytics on time-series data is essential for today’s financial services firms. This data, in turn, brings with it a host of opportunities and challenges: offering unmatched granularity for market analysis, on the one hand, but also requiring highly specific tools and data architecture to query, store, and analyze efficiently.
In recent months, Snowflake has paid close attention to both the utility and complexity time-series data—including supermassive volumes of TICK historical data—brings to financial service businesses. Their response to this thorny intersection of necessity and toil has been to do what they do best, delivering advanced time-series capabilities from right there in the Snowflake AI Data Cloud. Snowflake’s scalable, single-source-of-truth architecture, in turn, empowers analysts to focus on strategy rather than infrastructure, making it a key enabler for improving market intelligence and operational performance.
This blog will take a look at some of the advanced functionality Snowflake has been bringing to market in the last six months, dialing in on what they mean for financial services and insurance businesses in particular. We’ll also look at how Hakkoda and Snowflake are partnering to help deliver powerful analytics solutions in the cloud, including a look at a joint customer’s journey out of its legacy data swamp and into new revenue opportunities.
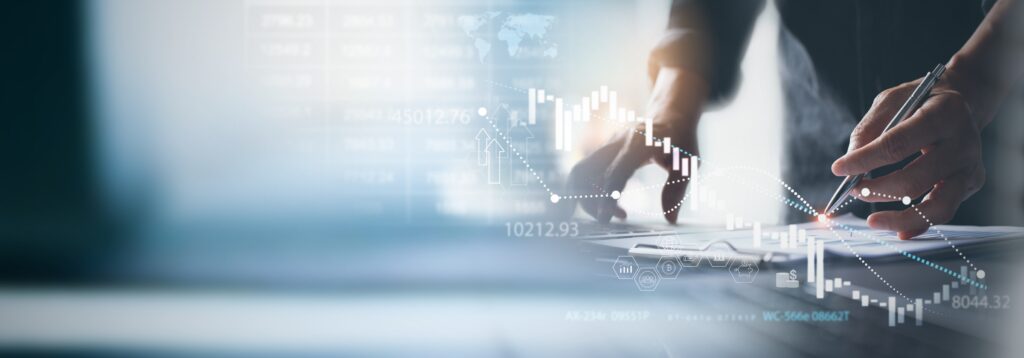
Unique Time-Series Functions in Snowflake
Snowflake has developed several purpose-built functions to streamline time-series data processing, enabling financial analysts to extract meaningful insights from large, complex datasets. Key functions include:
- TIME_SLICE: This function allows users to group timestamped data into uniform intervals (e.g., every minute, hour, or day). By standardizing time buckets, TIME_SLICE simplifies the evaluation of trends, volatility, and performance over fixed periods—crucial for identifying patterns in market behavior or operational metrics.
- ASOF_JOIN: Designed for joining two time-series datasets where exact timestamps don’t align, ASOF_JOIN matches records based on the closest prior timestamp. This is especially useful in financial applications like matching trades with quotes or aligning economic indicators with market events, ensuring consistent, lag-aware analysis.
- RANGE_BETWEEN: Often used in window functions, RANGE_BETWEEN defines a flexible frame for analytical queries based on value ranges or time intervals. Analysts can calculate running totals, averages, or deltas across rolling windows—ideal for monitoring intraday price shifts or volume changes.
These native time-series capabilities make Snowflake an efficient, performant, and scalable platform for handling financial datasets like TICK data, helping firms move from raw streams to actionable insights with less overhead and faster performance.
Optimizing Time-Series Data with Hakkoda and Snowflake
Of course, effectively leveraging time-series data in Snowflake requires more than just access to the platform. It also demands thoughtful architecture, domain-specific data modeling, and a clear understanding of analytical goals. For financial services organizations, this often includes working with complex, high-volume datasets like TICK data, portfolio valuations, or intraday transaction records—all of which can be difficult to effectively migrate, enrich, and analyze.
Hakkoda, an IBM company, is a strategic Snowflake partner that supports financial institutions by helping them design and implement solutions that maximize the value of Snowflake’s native time-series capabilities. With experience in both modern data infrastructure and financial services workflows in particular, Hakkoda is uniquely equipped to help firms ensure time-series datasets are optimized and analytics-ready within Snowflake without losing focus on downstream business objectives.
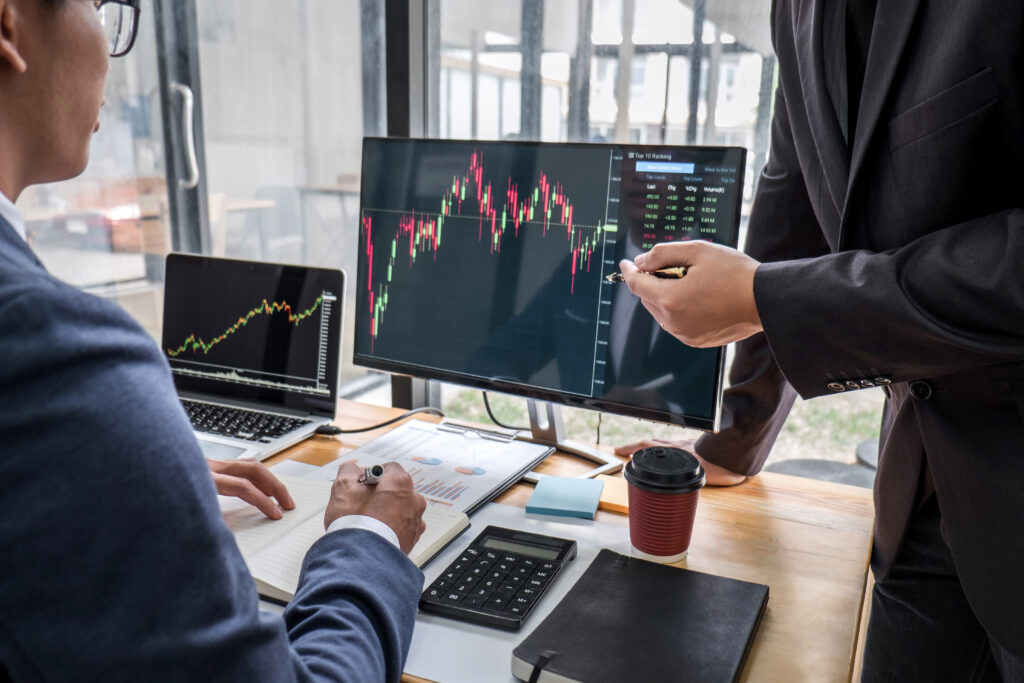
Time-Series Success in Action: A Customer Success Study
Consider the case of a global fixed income firm faced with a costly, on-premise TICK data swamp and legacy ETL bottlenecks. The firm understood it would need to modernize its analytics environment to retire growing storage and compute costs, but it also knew it could not risk compromising on performance or accuracy.
Over the course of a two-month proof of concept, the Hakkoda team successfully replicated its KDB-based time-series analytics using Snowflake, laying the groundwork to retire its legacy infrastructure. The migration not only reduced storage costs by approximately 60%, but also delivered compute efficiency gains while replicating the firm’s existing data model and Refinitiv dataset in Snowflake.
Leveraging Snowflake’s native capabilities—like TIME_SLICE, ASOF_JOIN, and range-based trend analysis—the firm enhanced its trade cost analytics and unlocked faster, more scalable insights. The initiative delivered results 9x faster than typical industry timelines, showing how the right combination of technology stack and data expertise can change the way enterprises think about their time-series datasets.
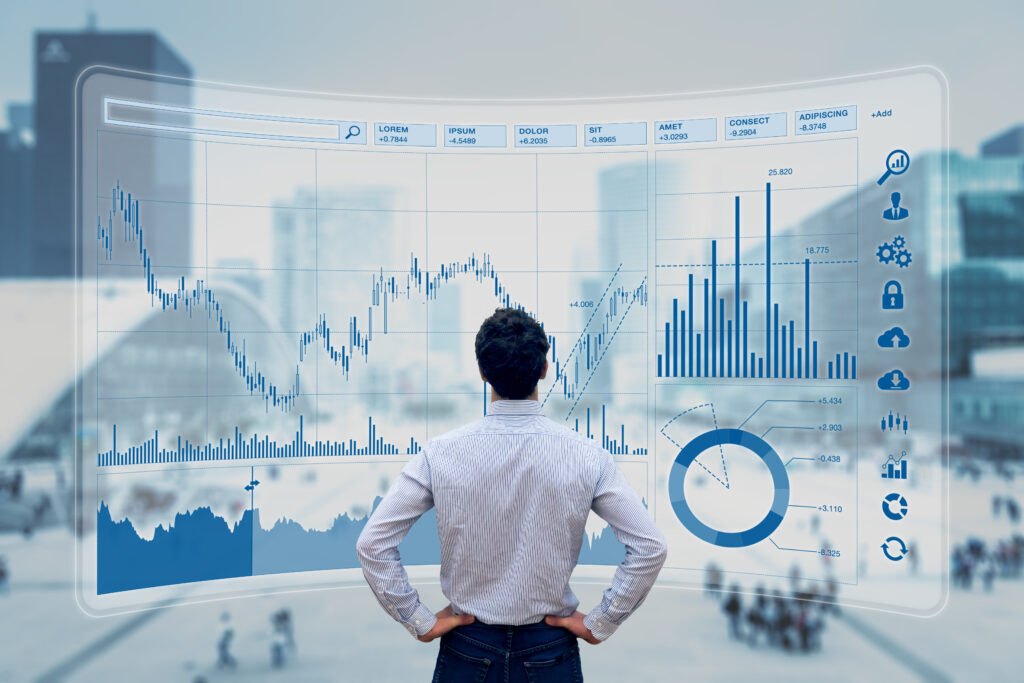
Conclusion: Achieving Competitive Edge with Time-Series Analytics
In financial services, making sense of time-series data is key to better decisions and performance. While many enterprises are still limping up that hill on the back of legacy technologies, Snowflake’s native functions—like TIME_SLICE, ASOF_JOIN, and RANGE_BETWEEN—show us that a new, more streamlined approach to time-series analysis is possible.
And not just possible, either. As the success story above illustrates, these modern time-series solutions open the door to more performant, cost-effective, and scalable analytics. And as TICK data providers, including Factset, ICE and BSLL, continue to add their data apps and listings to the Snowflake Marketplace, the opportunities awaiting financial service enterprises keep getting more expansive.
By moving away from legacy systems and leveraging modern data infrastructure, together with a data partner that can keep up with both industry and technical demands, financial institutions can turn some of the biggest, most granular datasets on the market into a measurable competitive advantage.
Ready to unlock a more cost-effective and performant approach to time-series analytics? Talk to one of our financial services data experts today.