Machine learning (ML) is changing the game in healthcare, but let’s face it—deploying and managing ML models at scale in a hospital or clinic can be a bit of a headache. Enter MLOps, the superhero that takes all the complexity out of machine learning and turns it into something smooth and efficient. By using MLOps and tools like Gitlab and Azure Dev Ops, healthcare organizations can get more reliable results, save time, and help patients faster—all while making sure everything is compliant and secure.
Healthcare is full of data—tons of it, from patient records to test results to notes scribbled by doctors. The problem? This data is often messy, hard to manage, and it takes a lot of time to make sense of. That’s where MLOps comes in. It automates all the behind-the-scenes work to get ML models into action quickly and keep them running smoothly. It’s like setting your favorite playlist on repeat—everything just flows!
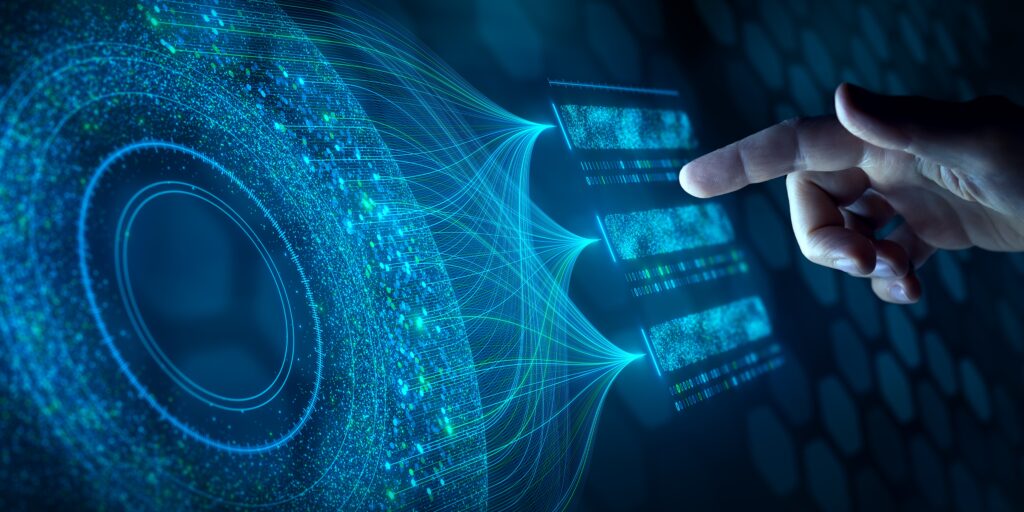
Why MLOps is a Game-Changer for Healthcare
Here’s why MLOps in healthcare is essential for smarter operations and outcomes:
- Handling Crazy Complex Data: Healthcare data is all over the place—clinical notes, patient histories, medical imaging, you name it. MLOps helps clean up and organize all that data so that machine learning models can actually do their job. MLOps is especially powerful in this respect when combined with a powerful, scalable cloud platform like the Snowflake AI Data Cloud, which acts as a single source of truth for your organization’s data and helps make sure your models are trained on high quality, accurate information.
- Keeping Models Up-to-Date: Unlike other industries, healthcare data keeps changing. MLOps keeps an eye on model performance, so if something isn’t working, it can be fixed in real-time.
- Staying Compliant: Regulations like HIPAA make healthcare one of the most heavily regulated industries. MLOps makes it easy to stay on the right side of the law by keeping track of every little thing that happens with data and models.
The Downstream Impact of MLOps in Healthcare
MLOps is making an impact in a lot of key areas, including some of healthcare’s biggest challenges:
- Predicting Patient Needs: Hospitals and doctors are using ML to predict which patients are at risk of complications or readmission. With MLOps, these models stay up-to-date with the latest patient data, so they’re always accurate and ready to help in real-time.
- Improving Medical Imaging: Radiologists rely on AI to spot problems in X-rays, MRIs, and CT scans faster and more accurately. MLOps ensures these models get updated as new imaging data rolls in, so the AI stays sharp and helps spot even the smallest issues.
- Clinical Trials Made Easier: Recruiting patients for clinical trials is tough. MLOps helps automate the process by matching eligible patients to the right trials quickly. That means faster trials and more people getting access to cutting-edge treatments.
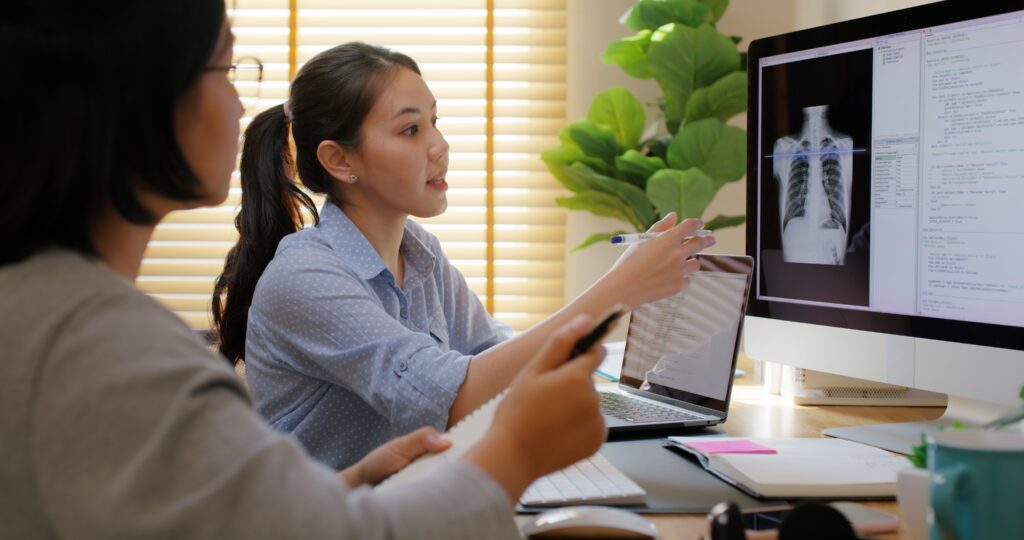
Why Healthcare Loves MLOps
So, why is MLOps the new best friend of healthcare? Here’s why it’s getting so much love:
- Faster and More Efficient: No more spending days getting models into action. MLOps automates the boring stuff, so teams can focus on what matters: improving patient care.
- More Accurate Predictions: MLOps ensures that models are constantly being tweaked and improved to give the most accurate results, which is especially important when it comes to things like diagnosing patients.
- Scalability: Want to roll out ML models across several hospitals? MLOps makes it easier to scale up without sacrificing quality.
- Better Collaboration: Data scientists, IT teams, and clinicians all work together to make sure the ML models are doing their best work, leading to better results for everyone.
The Challenges of MLOps in Healthcare (And How to Beat Them)
Of course, it’s not all sunshine and rainbows. Here are a few challenges healthcare faces when adopting MLOps:
- Data Privacy Worries: MLOps has solutions like encryption and privacy measures to make sure sensitive data stays safe.
- Changing Mindsets: Getting the whole healthcare team on board with MLOps can be tricky. But with the right training and education, everyone can start seeing the benefits.
- Resources and Budget: Setting up MLOps takes time and money, which can be a challenge for smaller practices. But once it’s up and running, the benefits pay off.
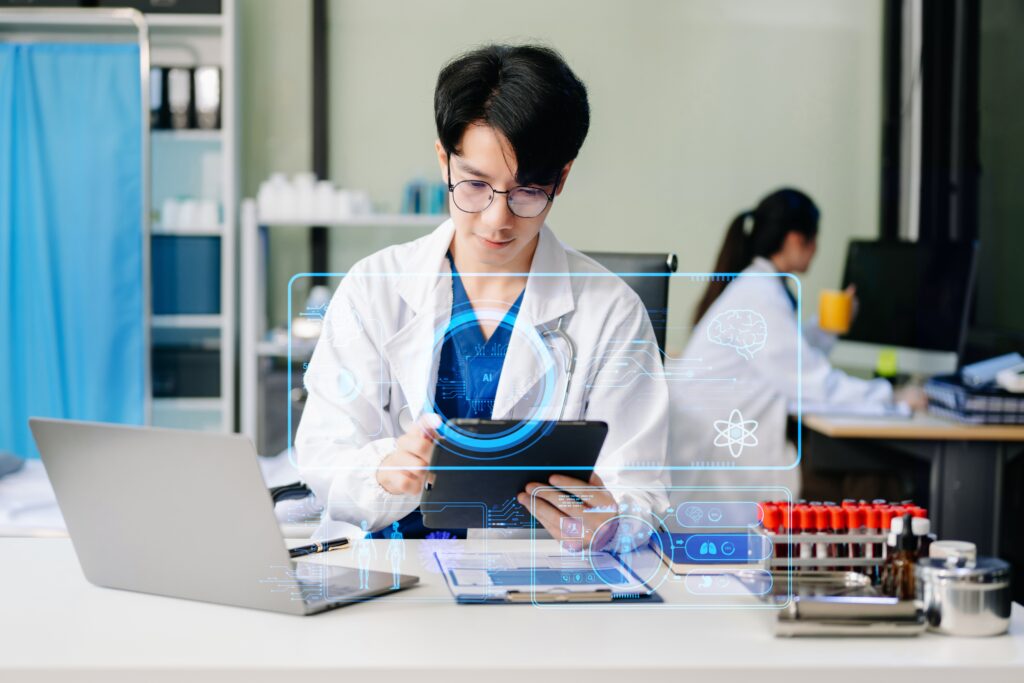
What’s Next for MLOps in Healthcare?
Looking ahead, MLOps will continue to be a game-changer in healthcare. Here are a few trends to watch:
- Edge Computing: Imagine real-time feedback from wearable devices or health sensors that help doctors make immediate decisions. That’s what edge computing and MLOps can do.
- AI That Makes Sense: Sometimes AI feels like a black box—especially in healthcare. MLOps will help make AI more transparent, so clinicians understand why a model is making a certain prediction.
- Personalized Medicine: As genomics and precision medicine continue to grow, MLOps will play a big role in maintaining the ML models that help tailor treatments to individual patients.
MLOps is changing the way healthcare runs. By taking care of the technical stuff behind the scenes, it makes machine learning more efficient, accurate, and scalable. As MLOps continues to evolve, it’ll unlock new possibilities for innovation, better care, and smarter healthcare.
Ready to explore how MLOps can help you scale your machine learning initiatives and drive patient-first innovation? Let’s talk today.