In the first part of this series, we talked about why data is the fundamental building block of embracing new AI technologies and issued the evergreen reminder that “Garbage In, Garbage Out” remains a crucial principle for AI implementation.
This piece will pick up where we left off, focusing on what you do after your data has been organized and prepared for analysis.
Put another way, we will answer the question: how do I use my data, together with AI, to drive my business goals and objectives?
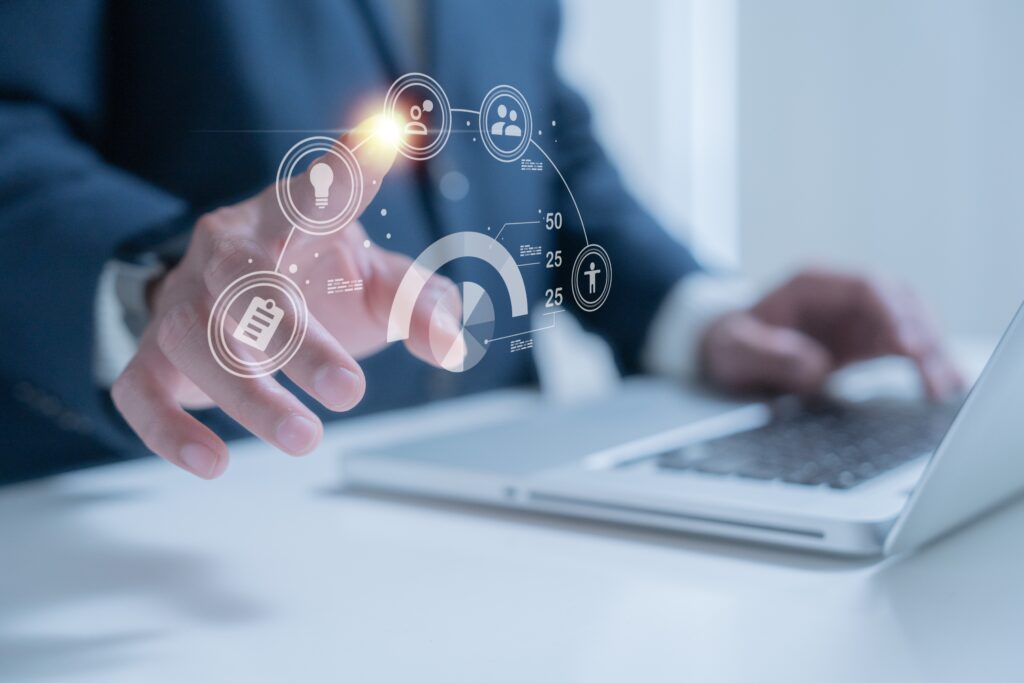
An AI Strategy Should Always Begin with the Data
Getting your data in order to be able to use advanced analytics to drive business outcomes will help you understand what data you already have.
In many cases, you will find data scattered across your organization you did not realize you had.
You will also be able to gain a more comprehensive view of your company, including assets, operational strengths, and even gaps or areas of improvement.
This process will give you baseline knowledge of the data assets available to you and can be paired with your business context, goals, and objectives to set up for analytic questions.
Addressing Business Needs with Analytics Questions
A common mistake organizations make when diving into AI and other emerging technologies is to start building and implementing without a real purpose. That is to say, building AI just to be able to boast you have done it.
You might also say that you need AI because your competitor has it and you cannot risk falling behind. But there’s more to successful AI implementations than just following the hype.
In practice, you want to take a more strategic approach to maximize the value your organization can get from AI.
The best place to start is creating Analytic Questions, similar to research questions, that are tied to your business objectives and future goals.
These questions will help to drive your research, analytics, and action plan for business outcomes. Remember that they are not necessarily AI questions. They are business questions that you can use as the north star for your AI implementation.
Here are some examples of what those questions might look like:
- How can we reduce the time to decision on legal contracts to innovate faster and reduce operating costs?
- How can we improve our customer experience to improve our customer retention?
- How can we improve our online customer acquisition process to reduce customer acquisition cost?
- What markets are we not reaching now that provide growth opportunities?
These are just a few examples, but notice they are focused questions based on business needs.
These questions provide a narrow starting point for exploring AI solutions to the problems. A focused question allows you to measure improvement, ROI, and other key metrics that matter to your business.
If the question is broad—such as, “where can we use an AI chatbot?”—it will be difficult to measure the value that a chatbot actually provides to your organization.
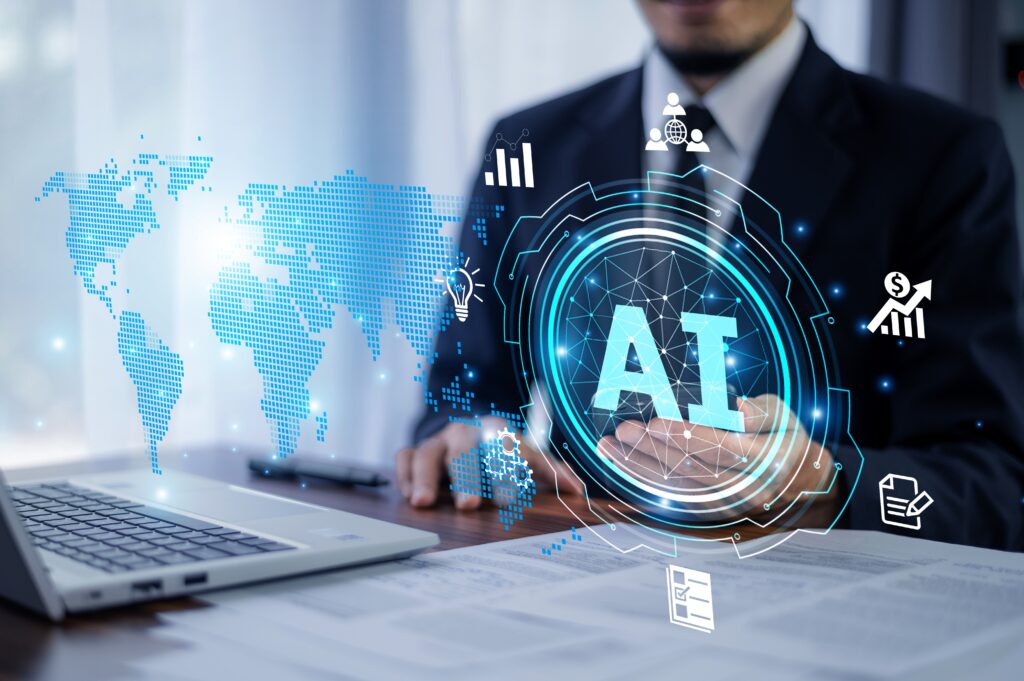
Identifying the Right AI Solution Starts with Identifying the Right Problem
If done correctly, your questions provide a starting point for a focused AI implementation.
There are many AI tools available for experimentation, and having a clear idea what problem you are trying to solve will help narrow down your search to applicable AI tools.
If I were given the first question above, here are some solutions I may explore.
What is the cause of the long lead time?
- Is it difficult to find the correct information or contacts in the organization to make an informed decision on the contract? If so, a chatbot trained on internal legal documents and policies may help employees find answers quicker.
- Is the long lead time due to a long backlog of contracts that need to be decided and there is too much volume for employees to clear out? If there is a large volume of historical decisions a machine learning model could be trained on previous decisions and automatically flag decisions for employees to review.
The analytic question sparked new questions that helped me think more about the problem. In practice you would make sure all your follow up questions are answered to ensure you are building the correct solution for your business.
There were different analytic tools that could be used to solve the same problem, it is not a clear one size fits all solution.
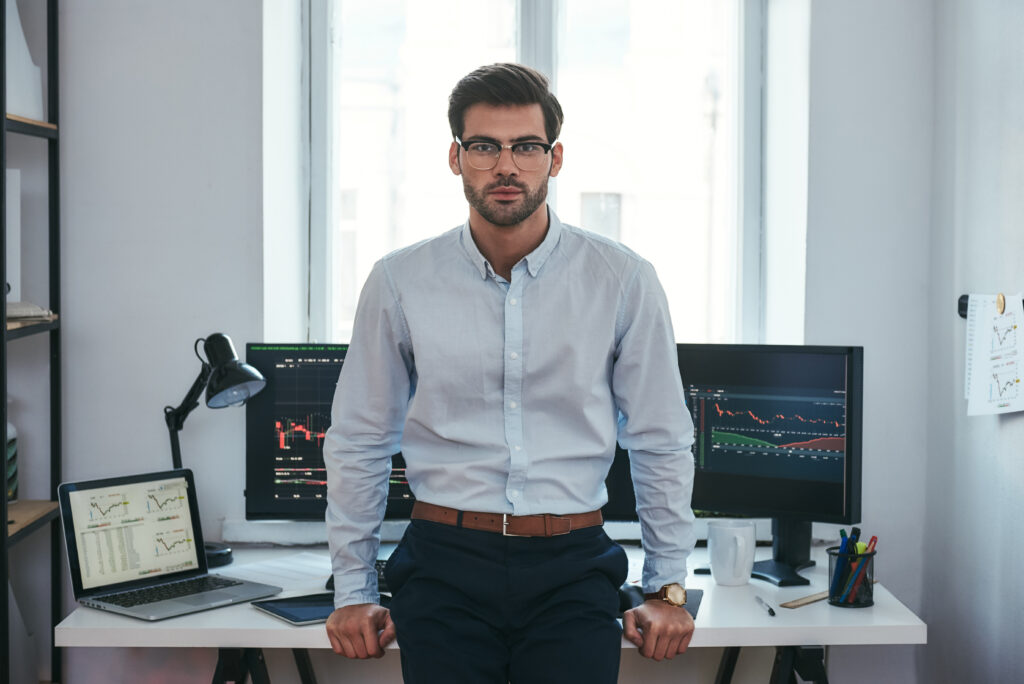
From AI Solutions to AI Return on Investment
Generative AI is a popular technology, but it may not always be the best solution. Try not to get tied down to one solution right away without exploring how different options can solve your problem.
If you do decide that AI is the best path forward, you can next begin to ask the age-old question of whether it’s best to build or buy that AI solution.
As you experiment you may discover one is easier and faster, one is too expensive, or maybe neither one performs well in validation and you need to explore another option.
Actively leveraging your data will allow you to move from passive analytics to describe your data to predicting future outcomes with your data to prescribing new business processes that allow you to reach your objectives.
This shift from collecting data to using it to answer business problems is an invaluable step in transitioning your data from a novelty to an asset. Data can help to inform the questions you need to ask about your business and analytics can guide answers.
Ready to deliver better return on your next AI investment while letting your data help guide the future of your business? Talk to one of our experts today.