Generative AI: It’s the siren call that’s been sounding for months, sending shockwaves through the business world and offering one of those rare periods of technological advancement that separate the early winners from the early losers. For data leaders, this shakedown has been particularly exciting to watch, in large part because a business’s success deploying generative AI and LLMs tends to hinge on how effective they were at building and maintaining a robust data framework in the first place. To leverage an LLM or generative AI instance, a company needs effective measures for storing, filtering, and protecting data.
As a result, the past few months have been a litmus test in data literacy for many industries, as business leaders, data scientists and other stakeholders set out to prove what they can, and sometimes unwittingly, can’t do with generative AI.
The good news for data consultancies and cloud-based providers like Snowflake? Your services just became more important than ever, and you’ve got a leg up on preparing your business to meet the AI revolution.
The Quick and Dirty on Generative AI
Generative artificial intelligence (AI) refers to algorithms that can be used to do everything from generate text, to code, images, and videos. Input some source material and wait for your algorithm to spit something out. Algorithms themselves are nothing new, but the business world has been waiting a long time for artificial intelligence to make a significant leap in quality, and that day has finally arrived.
ChatGPT is perhaps the most well-known recent development in generative AI: A large language model (LLM) that allows users to automate everything from writing an email to planning a trip itinerary. LLMs are a specific type of generative AI, and they represent a large chunk of the current opportunity across industries–take BLOOM or LLaMA, for example. The latter is Mark Zuckerberg and Meta’s attempt to enter the LLM-sphere.
If none of this sounds conceptually new to you, you’d be right. The shift that’s taken place over the last year hinges almost entirely on quality. We knew AI would take over routine tasks some day, so what’s the “revolution” everyone is so excited about?
According to Latanya Sweeney, professor of the Practice of Government and Technology at Harvard, “what makes generative AI different is that it seems to create something that didn’t exist before. As humans, we think of ourselves as the only ones creating. While generative AI is not creative in the way humans are, it is making the latest development in this technology feel really different.” In short, AI finally feels like it’s able to “create,” to “generate” something from virtually nothing. Put in some garbled instructions and you can get something polished and coherent back out.
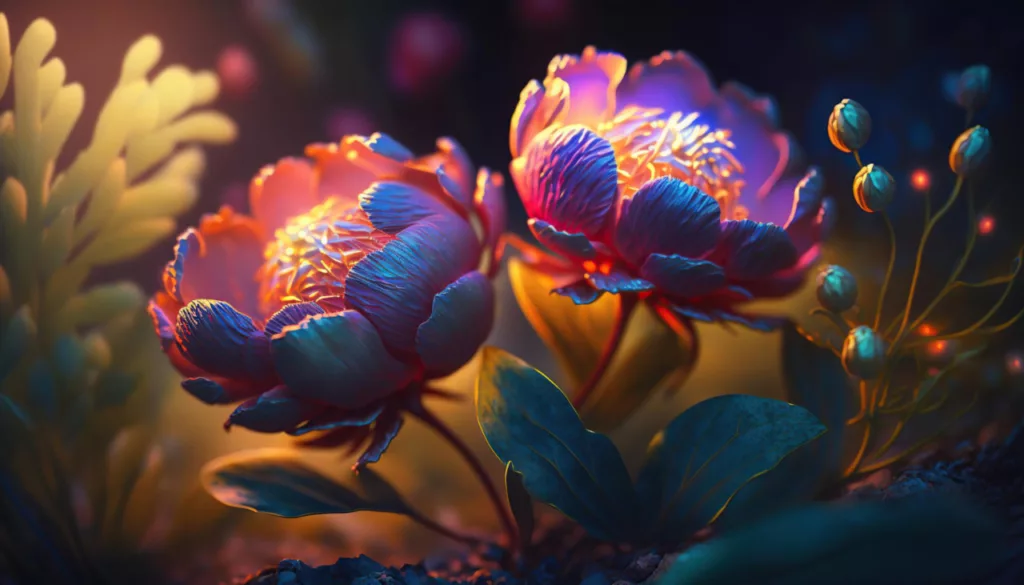
Where People Go Wrong with Generative AI
The potential of Generative AI for businesses, as you may have already imagined, is huge. What if entire teams of people could be winnowed down to one person feeding information into an LLM? What if entire portions of your company could be automated? Attendant to these musings is a fair amount of paranoia from people in professions like design, data entry, or paralegal. Will the need for human creativity and critical thinking all but disappear?
The short answer is no. The long answer is that generative AI is likely to place new and reduced value on certain types of activity. As any highschool teacher who has already had to grade a ChatGPT essay will tell you, there are still tangible limits to what generative AI can do and the benefits it can bring a business.
Where do companies fumble in their attempt to lean into generative AI and LLMs?
They Fail to Account for Data Needs & Implementation Costs
Unless you’re using a pre-trained LLM, most generative AI solutions take a considerable amount of time to train and deploy. This is because large language models require an incredible amount of processing power, specialized hardware, time and skilled personnel. Small and medium-sized businesses may not have the budget or infrastructure to create a tailored generative AI solution. Over-eager adopters have also already run into security issues. Whether it’s a result of failing to appropriately reign in their own employees or a lack of expertise when it comes to appropriately structuring AI components, some companies have already run headfirst into the wall that is security, data sharing, and successful implementation of generative AI.
They Imagine Generative AI as a One-Size-Fits-All Solution
Although tools such as ChatGPT are already making waves, it’s important to bear in mind that generative AI is still in its infancy. Currently, LLMs available in the market learn the surface form of the language and reproduce the most common (or the most highly probable) linguistic patterns.
If your business is looking to focus on specific tasks, such as answering questions on technology, you must train the model on a more specific set of information. Depending on how specialized your field is and how complex the tasks you want to automate with generative AI, training your LLM could require anywhere from weeks to months to do right, and like any meaningful data project, what accompanies that is thousands of hours of testing, evaluation, and building security controls that will help you keep your generative AI model up and running and certain pre-determined quality and security standards.
The Forget to Tune Their LLM
Tuning allows any large language model to improve its performance. As Prasad Thammineni notes, “pre-trained LLMs like GPT-3 and BERT are already powerful, but may not perform optimally for specific use cases right out of the box.” However, tuning a model too closely to the training data may result in overfitting. This can lead to errors and inaccurate predictions.
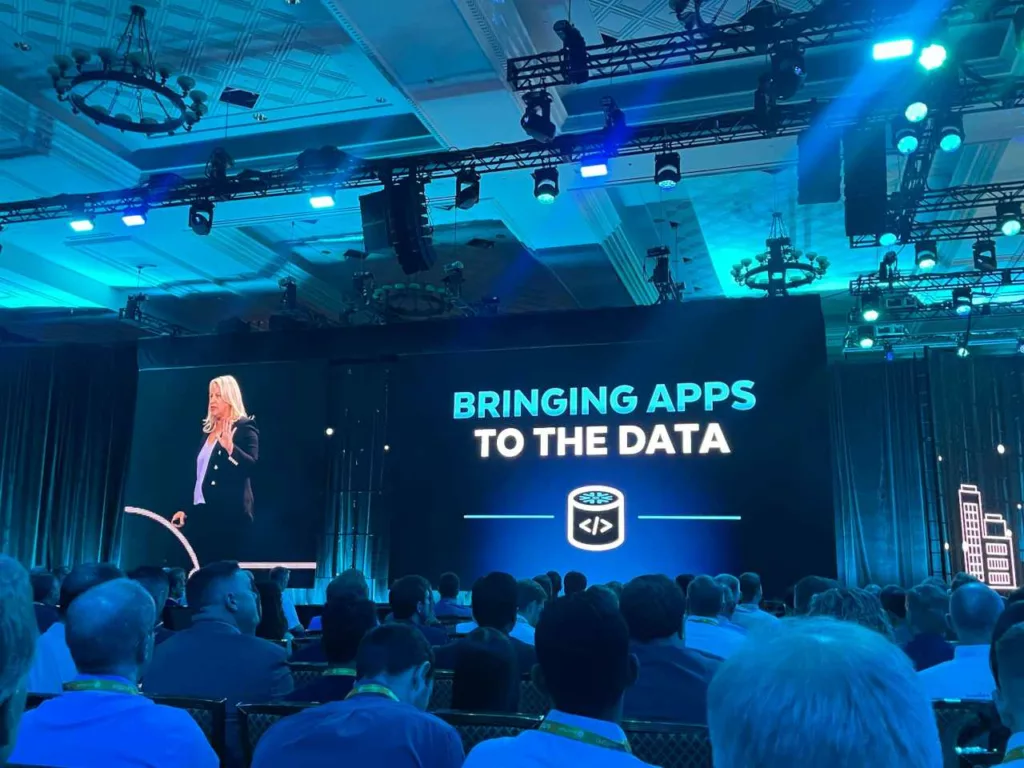
Who’s Winning with Generative AI & LLMs?
Snowflake, the Cloud Data Warehouse company, stated in their 2023 Data Trends report that “generative AI is prompting every industry to rethink core workflows and processes —catalyzing a fundamental shift across software and enterprises.” Many of the key advantages and uses for generative AI, especially in the world of data, are related to how processes are optimized. In short, how good is your data? How good is your data architecture? How good are you at training and deploying your generative AI? A good data strategy is the key to succeeding with generative AI and LLMs.
What do the early winners of generative AI have in common?
They Start with Great Data & Use Generative AI to Centralize Their Business
One of the key benefits of migrating to the modern data stack is the ability to store your data in a single, meaningful place. Using Snowflake, companies can “build pipelines to process data, training machine learning models, creating analytics queries, washboarding, and even powering entire applications.”
By having data in a single place, data experts can now leverage generative AI models to do more with it. Over the course of one year, for example, the number of jobs in the Data Cloud grew by 64%. This not only means that users are finding more ways to bring work with their data, it also implies that new technology is also increasing the amount of work being done.
They Embrace Automation
In January 2023, Snowflake saw a 71% rise in automated warehouse resize events when compared to that same month, in 2022. This simple example showcases how data teams (and companies) are implementing automation to enable greater efficiency and minimize operating costs. Generative AI is an important part of the automation trend.
The EnterprisersProject calls out generative AI as an important automation trend, along with resource optimisation, edge computing and DevSecOps.
Snowflake Introduces Generative AI Features
Yesterday, Snowflake announced its partnership with computing company Nvidia to enable customers in various sectors, such as finance, healthcare, and retail, to develop their own AI models using proprietary data. Nvidia’s NeMo platform, which facilitates training and running generative AI models, will be integrated into the Snowflake Data Cloud. The move aims to bring computing to the data rather than moving data to the computer, particularly for large amounts of valuable proprietary data.
The partnership is seen as a significant development, driven by the growing demand for AI strategies, including chatbot systems like ChatGPT. Nvidia, a major provider of AI hardware, has experienced substantial growth and reached a trillion-dollar valuation as a result. Snowflake’s Chairman and CEO, Frank Slootman, emphasized the importance of data in today’s landscape, stating that data is now eating software. The collaboration allows Snowflake users to leverage their own data for training new AI models, gaining a competitive edge while maintaining control over their valuable information.
While no financial details were disclosed, Nvidia stands to benefit from increased chip sales and the use of its Nvidia AI Enterprise software as more customers adopt computing solutions for AI-related tasks. The integration of Nvidia’s AI technology into Snowflake’s platform represents a crucial milestone in the field of data analytics, facilitating the seamless development and implementation of AI models directly on the data itself.
How Hakkoda Helps Companies Become Early Winners with Generative AI & LLMs
Recalling Colleen Kapase’s words in a previous keynote speech yesterday, “Innovation is a team sport and together we redefine what’s possible”. Her words resonate with Hakkoda’s spirit of collaboration, where we work together with industry partners to develop innovative solutions in different fields. Our data expert teams can guide you towards the successful implementation of generative AI solutions within your organization.
If you’re interested in innovating and streamlining your data pipelines state-of-the-art data technologies, contact us.