Last week, the AI in Healthcare & Life Sciences Leadership Summit brought together experts and leaders to explore the evolving impact of AI technologies on the provider, payer, medical device, and pharma spaces.
The cultural penetration of AI technologies, which according to one participant has reached 40% within just two years, showcases the rapid pace at which AI is being adopted in healthcare settings and points to the critical need for a measured, outcome-oriented approach.
This summit, subtitled “Innovative Solutions to Practical Problems,” served as a platform for innovators in the healthcare and life science industries to think about emerging technologies from a pragmatic point of view.
To that end, the event emphasized the transformative potential of AI in filling gaps in medical operations and streamlining processes, showcasing real-world examples of successful AI implementations in healthcare settings.
In this blog, we’ll highlight five of the biggest takeaways from those conversations.
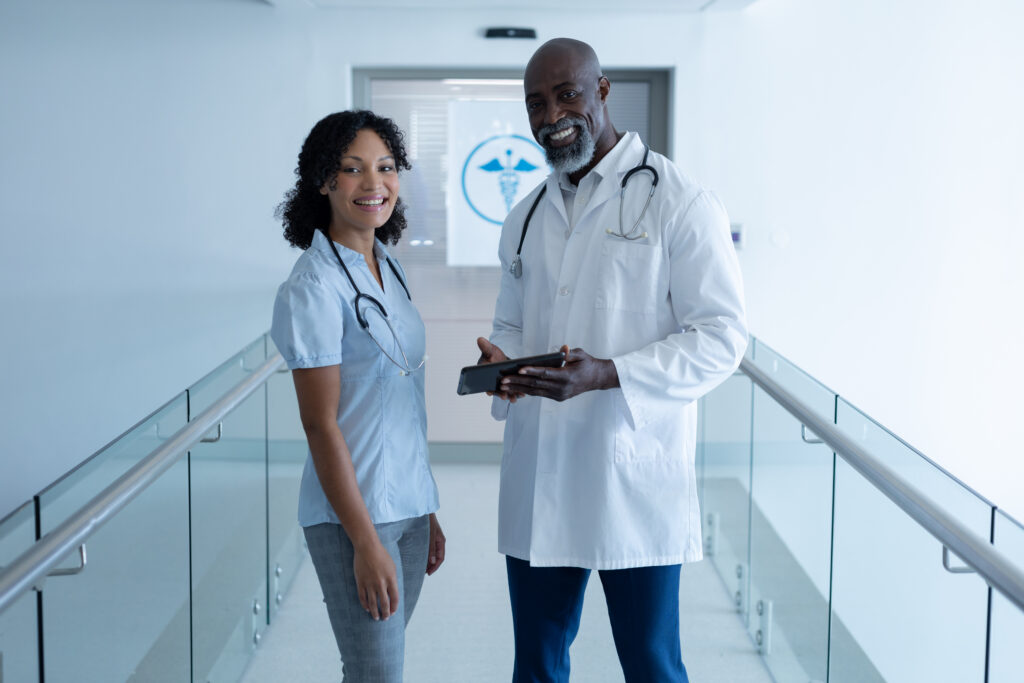
1. The Innovation-Production Gap
One of the most compelling frameworks shared over the two-day event was the juxtaposition of an “innovation factory” versus a “production factory.” While many organizations excel at creating rapid AI prototypes, these same organizations often struggle with the transition to scalable, production-grade solutions.
As one panelist noted, “Gen AI makes it super easy to prototype anything, but I see leaders making decisions like ‘one person did that in an hour, I don’t need a team’ and genuinely not understanding the gap to get to the product phase.”
This observation mirrors what we’ve experienced at Hakkoda when we sit down to talk AI with a client. In fact, it could be said that our healthcare AI implementation services are focused on bridging this exact gap. During the first day of the event, several key insights emerged about moving AI from innovation to production in healthcare. Those included:
- Starting with specific use cases rather than “doing AI” for its own sake.
- Investing in data foundations as the critical enabler of trustworthy AI.
- Rethinking organizational structures to support AI implementation at scale.
- Incorporating design thinking to ensure solutions integrate into clinical workflows.
- Establishing robust governance that covers both technical and ethical considerations.
2. The Data Foundation Challenge
Another recurring theme throughout the day was the critical importance of data foundations in AI implementation. One panelist noted that “for most organizations that want to start using AI, they want to use it to understand the data and do analytics off of it. But to be able to do that, we really need to have defined it very well.”
Another speaker cautioned: “Everyone thinks their structured data is already well-organized, and it’s almost always not. If you point Gen AI at a badly-curated data set, it’s going to give you back really bad results.”
An iterative approach to AI model development was emphasized, necessitating constant refinement and validation for reliability. The need for comprehensive data sets was highlighted, as well as the importance of cross-disciplinary collaboration to ensure models are both robust and clinically relevant.
Practical solutions, such as phased implementation and ongoing monitoring, were discussed to help healthcare organizations adapt to AI more seamlessly while building trust with key stakeholders.
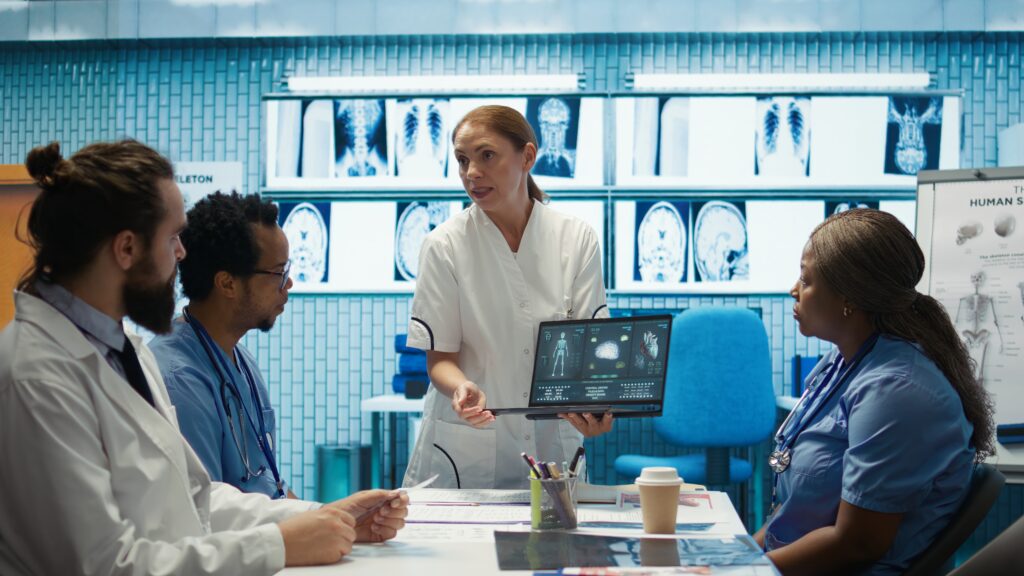
3. Success Stories: Where AI Is Delivering Value
Several clear trends emerged around successful AI applications in healthcare:
- Ambient Clinical Intelligence emerged as a game-changer for physician workflows. As the one health executive shared: “Ambient is the first time where the objective has just been ‘make the days easier.’ We have quotes from tons of physicians saying, ‘I get to go home on time. I get to go to my kids’ soccer games. I get joy back into seeing patients.’” This aligns closely with Hakkoda’s healthcare operations solutions, many of which have been built with the express mission of reducing administrative burden and returning clinicians to patient care.
- Prior Authorization Workflows represent another area of significant impact. Another speaker, hailing from a major children’s hospital, highlighted how AI streamlines “work that is manual, error-prone, and easy to kind of pour over to AI approaches.”
- Data Cataloging and Enrichment is also proving foundational. As one data.world speaker explained: “We’re using AI to help enrich metadata so that it has definitions. If we’ve cataloged your databases and understand what data is in that table, then we can define what that table might actually contain from a business definition perspective.” This AI-powered approach to data quality and enrichment also underscores an interesting pattern in the evolution of AI, where data foundations above result in more powerful AI models that can, in turn, be used to further improve data quality and governance measures.
4. Building a Community of Practice
Collaborative efforts were highlighted as essential for advancing AI in healthcare.
Speakers throughout the summit emphasized how technology providers, healthcare institutions, and academic researchers need to join forces if we are to genuinely push the boundaries of AI innovation.
One panel during the event was explicit that successful AI implementation is not just a technical challenge but a human one. As one panelist shared: “AI can’t possibly make the clinical decision that I can, given my training and depth and knowledge of my patients.” This underscores the need for thoughtful integration of AI into workflows and careful consideration of which use cases allow institutions to better leverage unique human skillsets.
Another speaker emphasized that “a diverse team matters and the team that is building this, the cross-functional team, is arguably more important than anything else from a trust and safety perspective.”
One particularly powerful anecdote shared was about a product that was nearly launched with a significant bias issue—tagging a person in a wheelchair as a “loser.” This stark example highlighted how critical diverse perspectives are in identifying blind spots before they become public relations disasters or ethical violations.
Many organizations are creating new roles to better enmesh a human element into AI processes. One panelist described how their organization has established positions for “foundational model researchers,” “AI-centered design researchers,” and “verification and validation specialists” to help ensure their AI efforts remain strategically—and humanely—aligned.
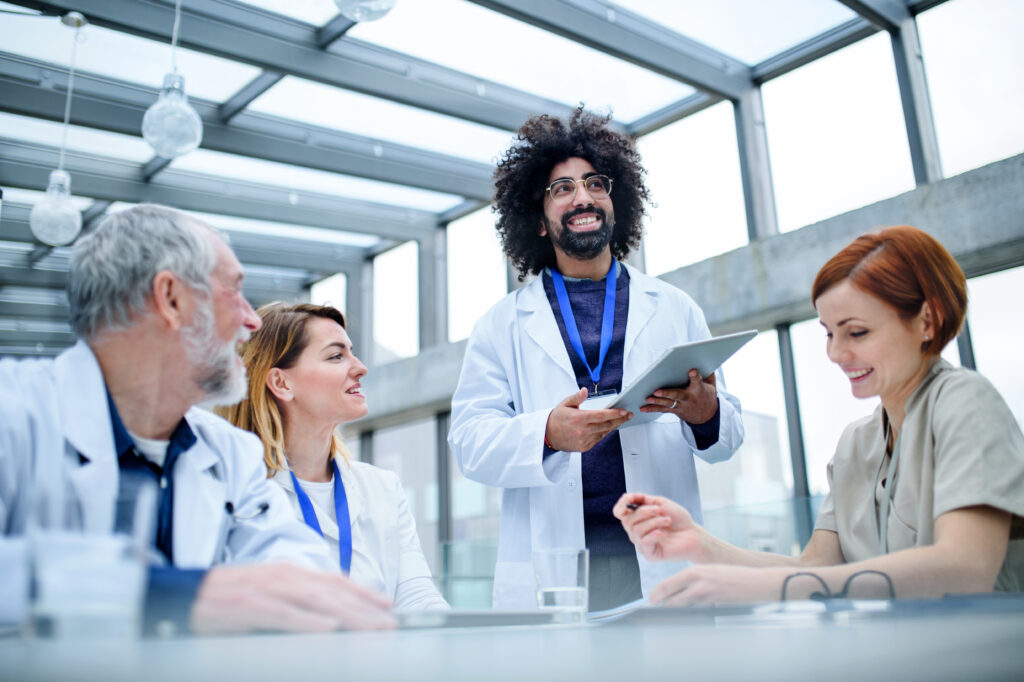
Looking Ahead with Clarity of Purpose
The AI in Healthcare Summit 2025 provided deep insights into how AI technologies are set to revolutionize the healthcare and life sciences sectors—at least, insofar as businesses continue to approach AI with unwavering clarity of purpose.
As we look to the future, it’s clear that AI in healthcare is no longer just aspirational. Organizations are getting their hands dirty with real implementations and driving real outcomes. But equally clear is the need for thoughtful approaches that balance innovation with unwavering focus and accountability.
The potential for AI to transform healthcare is enormous, from reducing administrative burden to enabling more personalized care, to addressing workforce shortages. However, realizing this potential will require continued focus on building diverse teams, measuring meaningful outcomes, ensuring secure and ethical use, and balancing short-term wins with long-term transformation.
At Hakkoda, we’re excited to continue partnering with healthcare organizations on this journey, bringing our deep expertise in both healthcare and data to help create AI solutions that truly advance the quadruple aim of better outcomes, lower costs, improved patient experience, and enhanced clinician satisfaction.
Ready to explore practical AI applications with the guidance of a trusted data partner who has been in your shoes? Talk to one of our experts today.